The Ultimate Guide to Data Annotation Tools and Platforms for Businesses
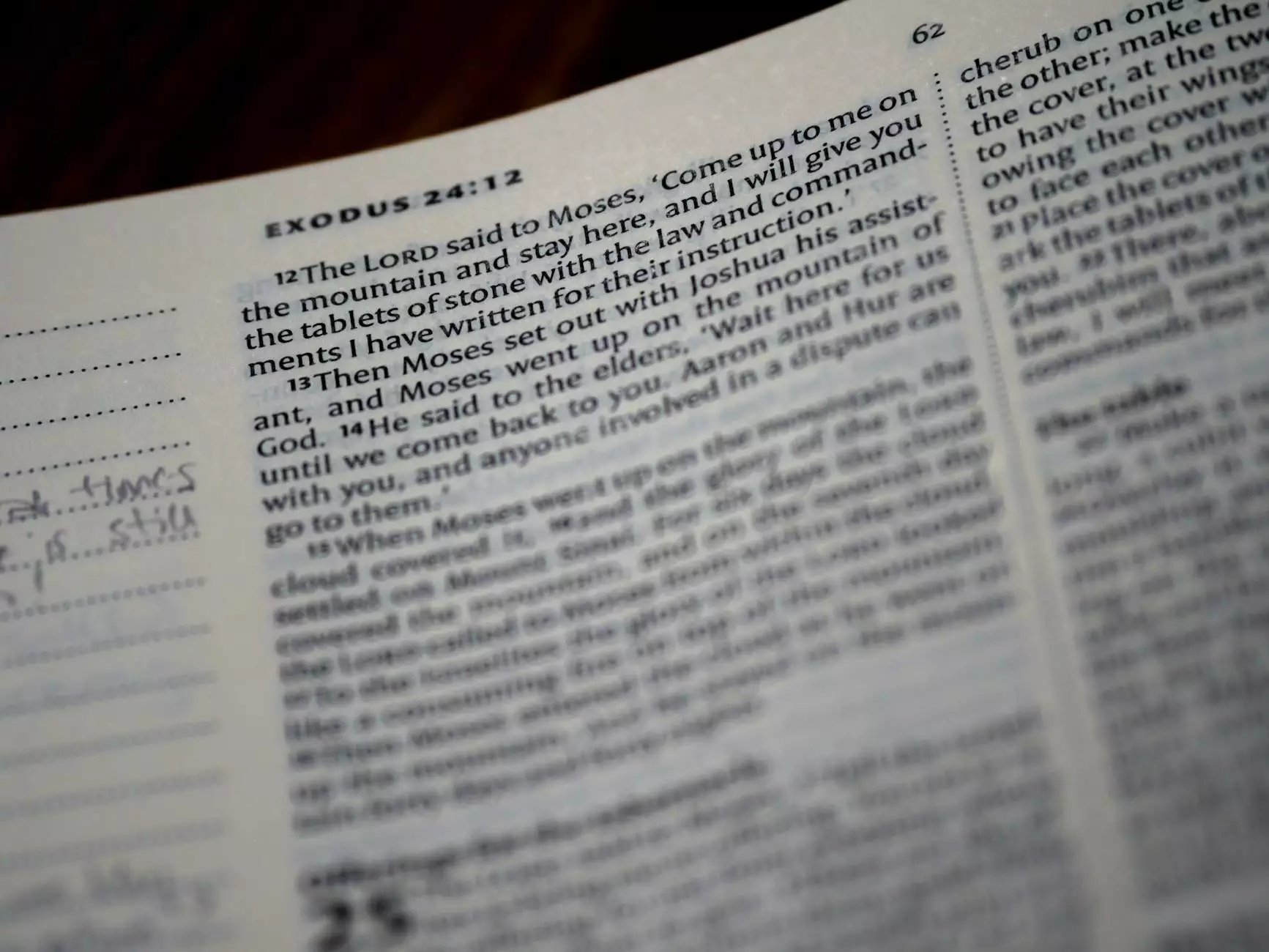
In today's data-driven world, the importance of data annotation cannot be overstated. Businesses are leveraging artificial intelligence (AI) and machine learning to drive innovation and efficiency. One of the most crucial steps in the AI development process is the ability to accurately annotate pictures and other data types. This article will explore the essential aspects of data annotation tools and platforms, focusing on their benefits, applications, and key considerations for businesses, particularly in the realm of annotating pictures.
Understanding Data Annotation
Data annotation refers to the process of labeling data to make it suitable for machine learning algorithms. This process includes:
- Image annotation: Labeling images for object recognition.
- Text annotation: Marking up texts to help machines understand them.
- Video annotation: Identifying events or actions within video frames.
- Audio annotation: Labeling audio clips for speech recognition.
Why Is Data Annotation Important?
The significance of data annotation lies in its role in training machine learning models. Here's why businesses should prioritize this aspect:
- Enhances AI accuracy: Quality annotations improve model performance.
- Facilitates automation: Well-annotated data sets can automate processes.
- Drives insights: Annotated data helps in extracting valuable business insights.
The Role of Data Annotation Tools
Data annotation tools provide the necessary environment for effectively annotating pictures and other data types. These tools can vary significantly in functionality and ease of use. Some of the primary features include:
FeatureDescriptionUser-Friendly InterfaceA simple, intuitive interface allows users to annotate efficiently without technical knowledge.Collaboration ToolsThese tools allow teams to work together seamlessly, sharing annotations and insights in real time.Integration CapabilitiesIntegration with existing systems and APIs enhances the functionality of data annotation tools.Machine Learning AssistanceSome tools employ machine learning to make suggestions or automate parts of the annotation process.Top Data Annotation Platforms in 2023
As businesses delve into AI and machine learning, an array of data annotation platforms have emerged to cater to varying needs. Here are some leading platforms:
1. Keylabs.ai
Keylabs.ai stands out in the realm of data annotation tools. It offers a comprehensive platform that allows businesses to annotate pictures with precision and speed. The tool supports various data types and is known for its robust collaboration features, making it ideal for teams.
2. Supervisely
Supervisely is an open-source platform that provides advanced image and video annotation capabilities. It caters to businesses looking for flexibility and control over their data annotation processes.
3. Labelbox
Labelbox offers an intuitive interface that's excellent for teams just starting out in data annotation. It features tools for annotating pictures and provides strong support for video and text as well.
4. Scale AI
Scale AI is known for its powerful API that can integrate seamlessly with other technologies. It's particularly effective for companies looking to handle large volumes of data quickly.
Best Practices for Annotating Pictures
When it comes to annotating pictures, following best practices can significantly enhance the quality of your annotations. Here’s what you need to know:
1. Define Clear Guidelines
Establish clear annotation guidelines to ensure consistency across your dataset. This includes:
- Label definitions: Define what each label means.
- Annotation tools: Outline the tools and methods for annotation.
- Quality standards: Set the bar for what constitutes a high-quality annotation.
2. Use Automated Assistance
Leverage tools with AI capabilities to assist in your annotation processes. Automated suggestions can speed up your work and help maintain consistency.
3. Quality Control Measures
Implement a robust quality control system to review annotations periodically. This ensures that the data remains reliable and relevant.
4. Continuous Training
Regularly train your annotators on updates in processes and tools. A well-trained team produces better-quality annotations.
The Future of Data Annotation
As technology evolves, so will the methodologies surrounding data annotation. Here are trends to watch for:
- AI-Powered Annotation: Increased use of artificial intelligence to automate and optimize the annotation process.
- Real-Time Collaboration: Enhanced platforms that allow real-time collaboration among remote teams.
- Multi-Modal Annotation: Tools that allow businesses to annotate various types of data (images, videos, audio) seamlessly.
Conclusion
In summary, the ability to annotate pictures and other data types effectively is pivotal for businesses aiming to harness the power of AI. By utilizing advanced data annotation tools and platforms like Keylabs.ai, businesses can ensure that their data is prepared for the future of machine learning. Investing in quality annotation processes will drive better outcomes, enhance automation, and ultimately lead to informed decision-making. The future is clearly data-driven, and staying ahead means embracing the best practices in data annotation.